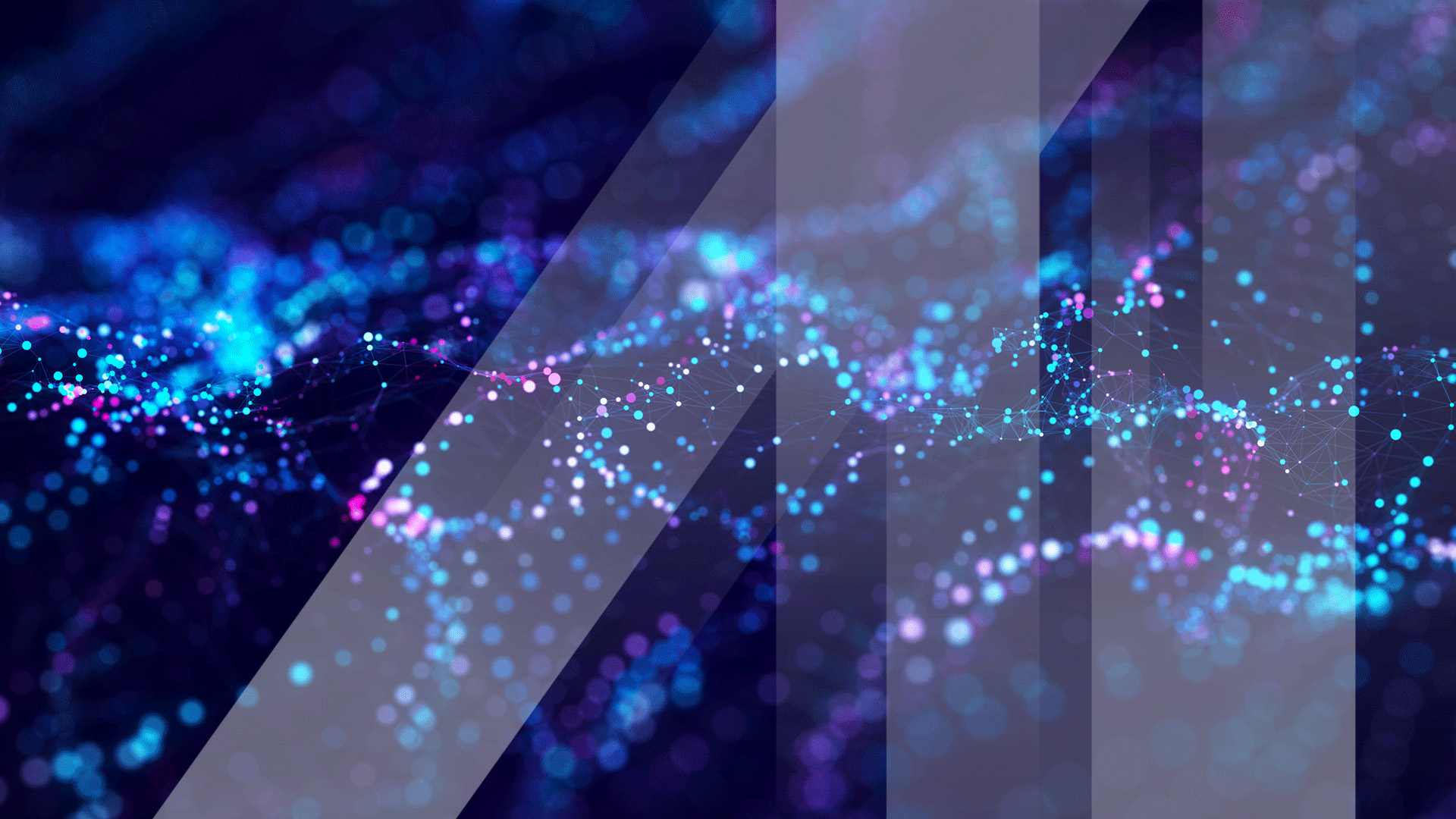
Artificial intelligence
Navigating the legal landscape
The AI regulatory landscape is changing. As the landmark EU AI Act sweeps into force, businesses need to consider their AI governance processes, ahead of the application in February 2025.
The regulatory framework for AI around the world is expected to continually evolve and grow in complexity.
Our AI hub will help you navigate AI risks, opportunities and regulation, and the legal implications, within the evolving legal landscape.
Featured insights
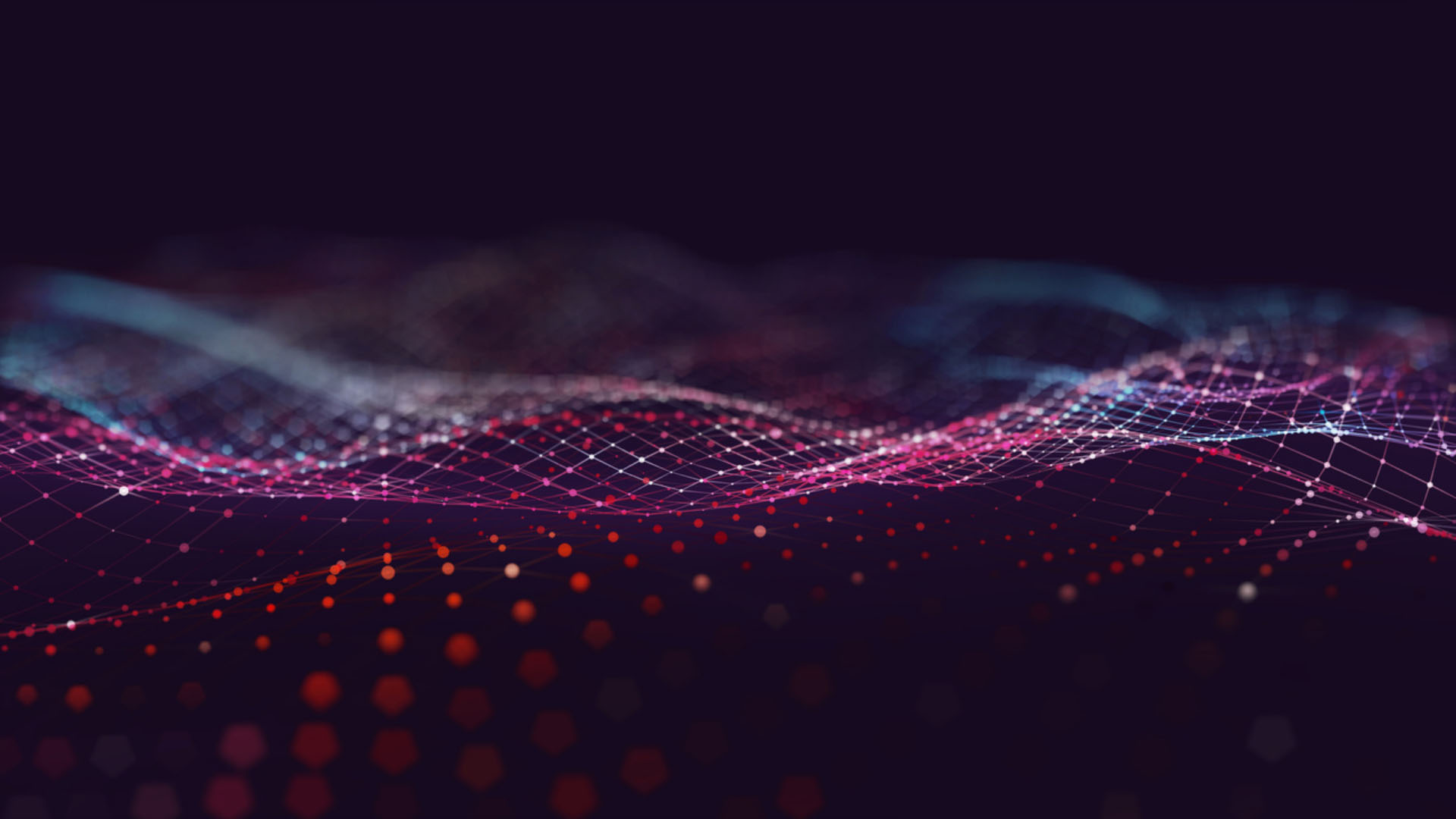
Subscribe to Inside Tech Law
Head of Information Governance, Privacy and Cybersecurity, EMEA
Email
marcus.evans@nortonrosefulbright.com
Global Head of Technology
Email
richard.zembek@nortonrosefulbright.com
Global head of FinTech
Email
sean.murphy@nortonrosefulbright.com
Senior Partner, Canadian Head of Technology and Canadian Co-Head of Cybersecurity and Data Privacy
Email
imran.ahmad@nortonrosefulbright.com
Partner, Co-Head of Cybersecurity
Email
christoph.ritzer@nortonrosefulbright.com
US Head of Technology and US Head of eDiscovery and Information Governance
Email
andrea.dambra@nortonrosefulbright.com