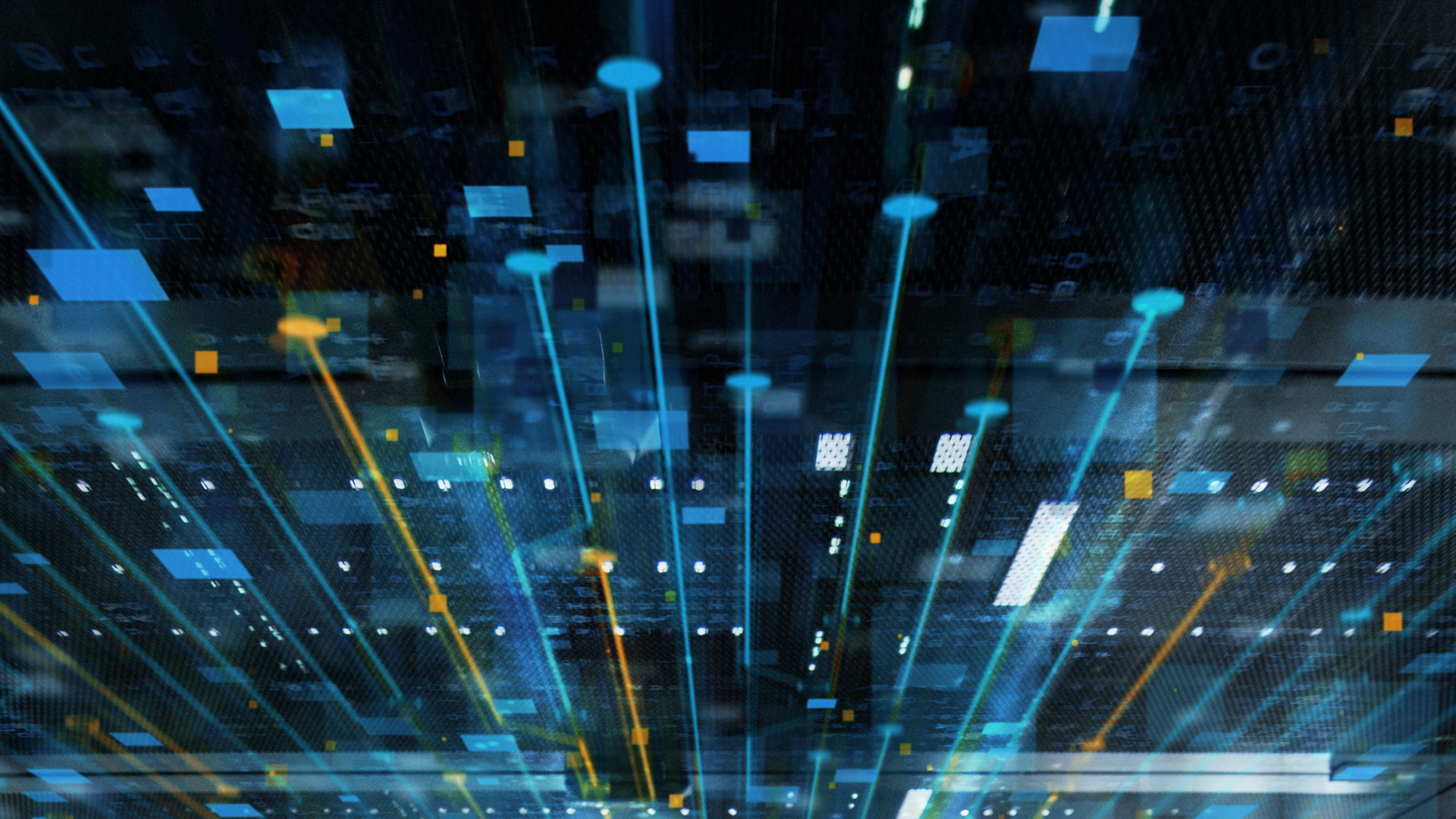
Artificial intelligence
Artificial intelligence (AI) is transforming the way we work, and as businesses shift more and more of their operations to AI technologies, there are some legal implications that must be considered including regulatory compliance and ethical, commercial, intellectual property and data privacy related issues. Regulation of AI is on the horizon, with the EU playing a leading role, which will impose significant obligations impacting businesses across many, if not all, sectors of the economy. Our AI hub will keep you up to date with the legal implications of using AI in your organization to help you navigate the evolving legal landscape.
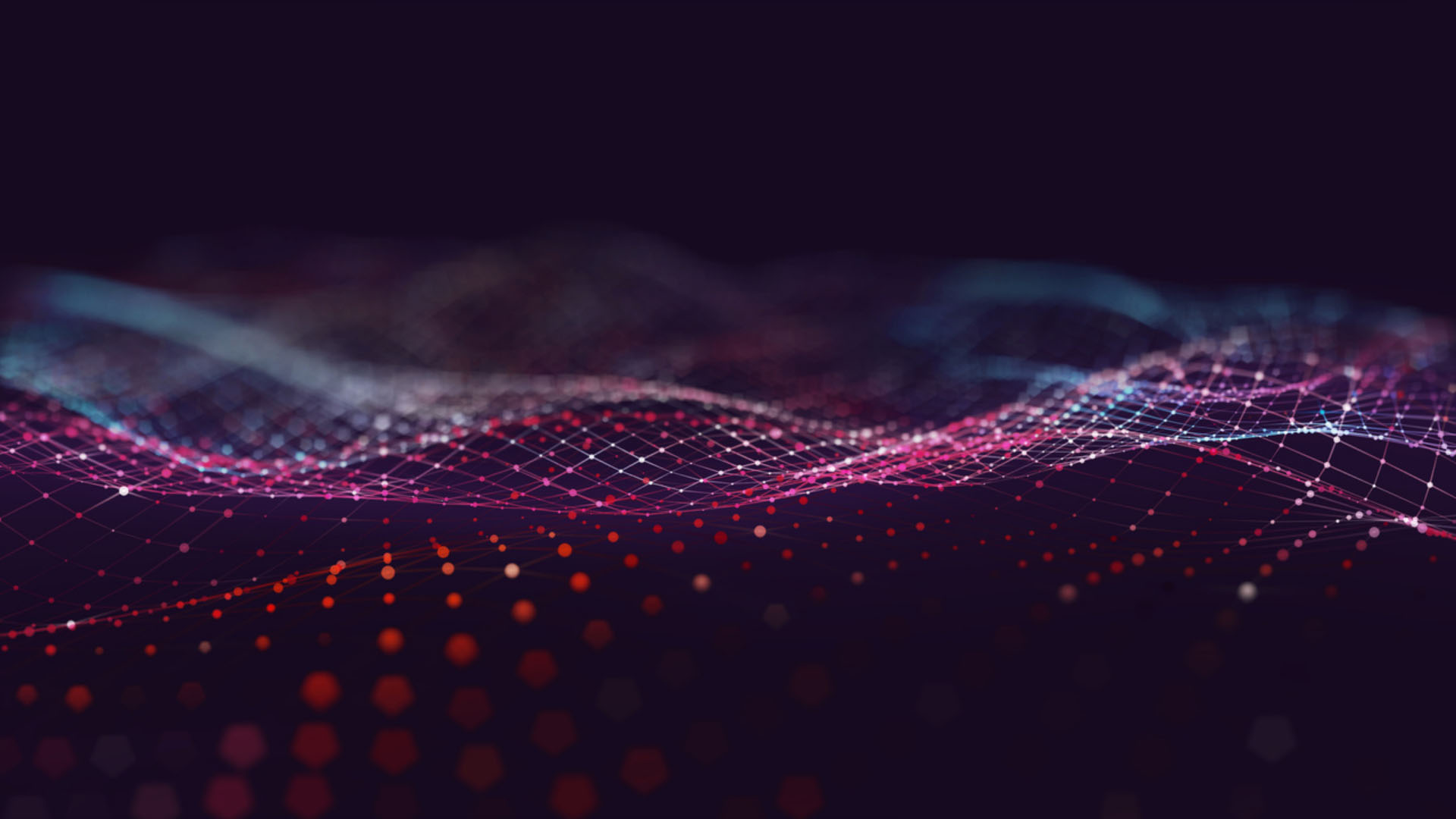